
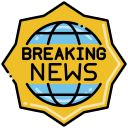
Tough-guy Ted “Bundy” Nugent also shat on himself to dodge the Vietnam draft.
Tough-guy Ted “Bundy” Nugent also shat on himself to dodge the Vietnam draft.
Why do bots
Suddenly appear? 🐦
That’s pretty disingenuous - it’s one of the many reasons that comprise a pattern of behavior whereby Microsoft makes Windows worse at each iteration. More bloat, more spying, more locked-down for user “security”. And for what? The dubious benefit of being “compatible” with other shitheel software providers like Adobe who use their monopoly power to stranglehold the corporate and professional media sectors? Toeachizown but IDK how anyone can use Windows by choice. The small amount I have to use it at work is torture enough.
I think it does look pretty cool. I applaud automotive design that dares to be different. Everything nowadays is a giant snarling grill with angry anime eye headlights up front, then a bunch of superfluous sharp creases and fake air vents to add visual elements for the sake of it. Tesla took a boldly minimalist approach with this one.
Before you crucify me, note that I don’t particularly like the vehicle overall - it doesn’t seem to be a design that translates well to mass production, practicality of maintenance, or pedestrian safety. It’s no Alfa 33 Stradale, but I think visual flare isn’t an area you can fault it much.
Rivian has done a good job of embracing EV design features (e.g. lack of need for frontal air intakes) in a more conventional way.
We tend to think of these models as agents or persons with a right to information. They “learn like we do” after all.
This is again a similar philosophical tangent that’s not germane to the issue at hand (albeit an interesting one).
I think you’ll see that if you only feed an LLM art or text from only one artist you will find that most of the output of the LLM is clearly copyright infringement if you tried to use it commercially.
This is not a feasible proposition in any practical sense. LLMs are necessarily trained on VAST datasets that comprise all kinds of text. The only type of network that could be trained on only one artist’s corpus is a tiny pedagogical tool like Karpathy’s minGPT https://github.com/karpathy/minGPT, trained solely on the works of Shakespeare. But this is not a “Large” language model, it’s a teaching exercise for ML students. One artist’s work could never practically train a network that could be considered “Large” in the sense of LLMs. So it’s pointless to prevaricate on a contrived scenario like that.
In more practical terms, it’s not controversial to state that deep networks with lots of degrees of freedom are capable of overfitting and memorizing training data. However, if they have other additional capabilities besides memorization then this may be considered an acceptable price to pay for those additional capabilities. It’s trivial to demonstrate that chatbots can perform novel tasks, like writing a rap song about Spongebob going to the moon on a rocket powered by ice cream - which is surely not existent in any training data, yet any contemporary chatbot is able to produce.
As far as science and progress, I don’t think that’s hampered by the view that these companies are clearly infringing on copyright.
As an example, one open research question concerns the scaling relationships of network performance as dataset size increases. In this sense, any attempt to restrict the pool of available training data hampers our ability to probe this question. You may decide that this is worth it to prioritize the sanctity of copyright law, but you can’t pretend that it’s not impeding that particular research question.
As far as “it’s on the internet, it’s fair game”. I don’t agree. In Western countries your works are still protected by copyright. Most of us do give away those rights when we post on most platforms, but only to one entity, not anyone/ any company who can read or has internet access.
I wasn’t making a claim about law, but about ethics. I believe it should be fair game, perhaps not for private profiteering, but for research. Also this says nothing of adversary nations that don’t respect our copyright principles, but that’s a whole can of worms.
We can’t just give up all our works and all our ideas to a handful of companies to copy for profit just because they can read and view them and feed them en masse into their expensive emulating machines.
As already stated, that’s where I was in agreement with you - It SHOULDN’T be given up to a handful of companies. But instead it SHOULD be given up to public research institutes for the furtherance of science. And whatever you don’t want to be included you should refrain from posting. (Or perhaps, if this research were undertaken according to transparent FOSS principles, the curated datasets would be public and open, and you could submit the relevant GDPR requests to get your personal information expunged if you wanted.)
Your whole response is framed in terms of LLMs being purely a product for commercial entities, who shadily exaggerate the learning capabilities of their systems, and couches the topic as a “people vs. corpos” battle. But web-scraped datasets (such as Imagenet) have been powering deep learning research for over a decade, long before AI captured the public imagination the way it has currently, and long before it became a big money spinner. This view neglects that language modelling, image recognition, speech transcription, etc. are also ongoing fields of academic research. Instead of vainly trying to cram the cat back into the bag, and throttling research, we should be embracing the use of publicly available data, with legislation that ensures it’s used for public benefit.
The advances in LLMs and Diffusion models over the past couple of years are remarkable technological achievements that should be celebrated. We shouldn’t be stifling scientific progress in the name of protecting intellectual property, we should be keen to develop the next generation of systems that mitigate hallucination and achieve new capabilities, such as is proposed in Yann Lecun’s Autonomous Machine Intelligence concept.
I can sorta sympathise with those whose work is “stolen” for use as training data, but really whatever you put online in any form is fair game to be consumed by any kind of crawler or surveillance system, so if you don’t want that then don’t put your shit in the street. This “right” to be omitted from training datasets directly conflicts with our ability to progress a new frontier of science.
The actual problem is that all this work is undertaken by a cartel of companies with a stranglehold on compute power and resources to crawl and clean all that data. As with all natural monopolies (transportation, utilities, etc.) it should be undertaken for the public good, in such as way that we can all benefit from the profits.
And the millionth argument quibbling about whether LLMs are “truly intelligent” is a totally orthogonal philosophical tangent.
I heard some of the really wealthy Silicon Valley types kit out their own private laboratory and hire chemists.
Since the forces that determine policy are largely tied up with corporate profit, promoting the interests of domestic companies against those of other states, and access to resources and markets, our system will misuse AI technology whenever and wherever those imperatives conflict with the wider social good. As is the case with any technology, really.
Even if “banning” AI were possible as a protectionist measure for those in white-collar and artistic professions, I think it would ultimately be unfavorable with the ruling classes, since it would concede ground to rival geopolitical blocs who are in a kind of arms race to develop the technology. My personal prediction is that people in those industries will just have to roll with the punches and accept AI encroaching into their space. This wouldn’t necessarily be a bad thing, if society made the appropriate accommodations to retrain them and/or otherwise redistribute the dividends of this technological progress. But that’s probably wishful thinking.
To me, one of the most worrying trends, as it’s gained popularity in the public consciousness over the last year or two, has been the tendency to silo technologies within large companies, and build “moats” to protect it. What was once an open and vibrant community, with strong principles of sharing models, data, code, and peer-reviewed papers full of implementation details, is increasingly tending towards closed-source productized software, with the occasional vague “technical report” that reads like an advertising spiel. IMO one of the biggest things we can lobby for is openness and transparency in the field, to guard against the natural monopolies and perverse incentives of hoarding data, technical know-how, and compute power. Not to mention the positive externality spillovers of the open-source scientific community refining and developing new ideas.
It’s similar to how knowledge of the atomic structure gave us both the ability to destroy the world, or fuel it (relatively) cleanly. Knowledge itself is never a bad thing, only what we choose to do with it.
I take your point, but in this specific application (synthetically generated influencer images) it’s largely something that falls out for free from a wider stream of research (namely Denoising Diffusion Probabilistic Models). It’s not like it’s really coming at the expense of something else.
As for what it’s eventually progressing towards - who knows… It has proven to be quite an unpredictable and fruitful field. For example Toyota’s research lab recently created a very inspired method of applying Diffusion models to robotic control which I don’t think many people were expecting.
That said, there are definitely societal problems surrounding AI, its proposed uses, legislation regarding the acquisition of data, etc. Often times markets incentivize its use for trivial, pointless, or even damaging applications. But IMO it’s important to note that it’s the fault of the structure of our political economy, not the technology itself.
The ability to extract knowledge and capabilities from large datasets with neural models is truly one of humanity’s great achievements (along with metallurgy, the printing press, electricity, digital computing, networking communications, etc.), so the cat’s out of the bag. We just have to try and steer it as best we can.
I have to disagree about that last sentence. Augmenting LLMs to have any remotely person-like attributes is far from trivial.
The current thought in the field about this centers around so-called “Objective Driven AI”:
in which strategies are proposed to decouple the AI’s internal “world model” from its language capabilities, to facilitate hierarchical planning and mitigate hallucination.
The latter half of this talk by Yann LeCun addresses this topic too: https://www.youtube.com/watch?v=pd0JmT6rYcI
It’s very much an emerging and open-ended field with more questions than answers.
In a sense… yes! Although of course it’s thought to be across many modalities and time-scales, and not just text. Also a crucial piece of the picture is the Bayesian aspect - which also involves estimating one’s uncertainty over predictions. Further info: https://en.wikipedia.org/wiki/Predictive_coding
It’s also important to note the recent trends towards so-called “Embodied” and “4E cognition”, which emphasize the importance of being situated in a body, in an environment, with control over actions, as essential to explaining the nature of mental phenomena.
But yeah, it’s very exciting how in recent years we’ve begun to tap into the power of these kinds of self-supervised learning objectives for practical applications like Word2Vec and Large Language/Multimodal Models.
Many modern theories in cognitive science posit that the brain’s objective is to be a kind of “prediction machine” to predict the incoming stream of sensory information from the top down, as well as processing it from the bottom up. This is sometimes referred to through the aphorism “perception is controlled hallucination”.
The Japanese SCMaglev only has the cooling stuff on the train, not along the entire length of the track.
And I think there is a “high-temperature SC Maglev” in development in China too.
Why no superconducting maglev tho?
I joined Twitter fairly recently as Machine Learning Twitter is/was a thing, and I wanted to stay abreast of news from people like Andrej Karpathy, Chris Olah, Andrew Ng etc., especially since r/MachineLearning went down the shitter.
But I can’t even - I log on and just instantly see ragebait posts from Daily Mail talking heads and bullshit.
Are there any better alternatives for this purpose?
I can’t help feeling a lot of these new motorized doodads are total deathtraps. I used to have a 40mph kaabo electric scooter and although it was mega fun, I sold it before it had a chance to kill me. Small wheels, poor geometry for stability, small caster angle, intoxicatingly high power - it’s a recipe for disaster.
Fair enough but it just seems like a fluffy distinction.
And I don’t think they “tweak the algorithm” so much as generate a load more training data of that one specific task to get it up to spec.
In any case, humans make mistakes on lots of stuff too, so if the criterion for “true” understanding is to make no mistakes then humans cannot be said to understand either.
I still don’t understand what you mean. If you don’t have a criterion for “actually” understanding, how has it demonstrably failed?
I used to use FL Studio, but hated using Windows. I got almost all features (including VSTs) to work in Ubuntu under Wine, but had a problem with WineASIO, which I seemed to require to use the USB sound card properly.
Because of that, I since changed to a DAW called REAPER which is built natively for Linux and works flawlessly and is very nice. There is a program called Yabridge to help run Windows VSTs. I even got more complicated plugins with authentication like Addictive Drums 2 to work using Wine no problem.
If you want a fully FOSS solution there is Ardour which is also great but a little less slick than Reaper IMO.